In recent years, the healthcare industry has witnessed a significant transformation driven by advancements in technology and the growing availability of data. Data science, with its ability to extract valuable insights from large and complex datasets, has emerged as a powerful tool for revolutionizing healthcare analytics. In this comprehensive guide, we will explore the role of data science in healthcare analytics, its applications, benefits, and challenges, and the transformative impact it has on improving healthcare outcomes.
Understanding Healthcare Analytics
Healthcare analytics involves the systematic analysis of healthcare data to extract meaningful insights and support decision-making processes. It encompasses a wide range of activities, including data collection, storage, processing, analysis, and interpretation, with the goal of improving clinical outcomes, enhancing patient care, and optimizing healthcare operations. Healthcare analytics leverages various types of data, including electronic health records (EHRs), medical imaging, genomic data, administrative claims, and patient-generated data, to generate actionable insights and drive evidence-based decision-making.
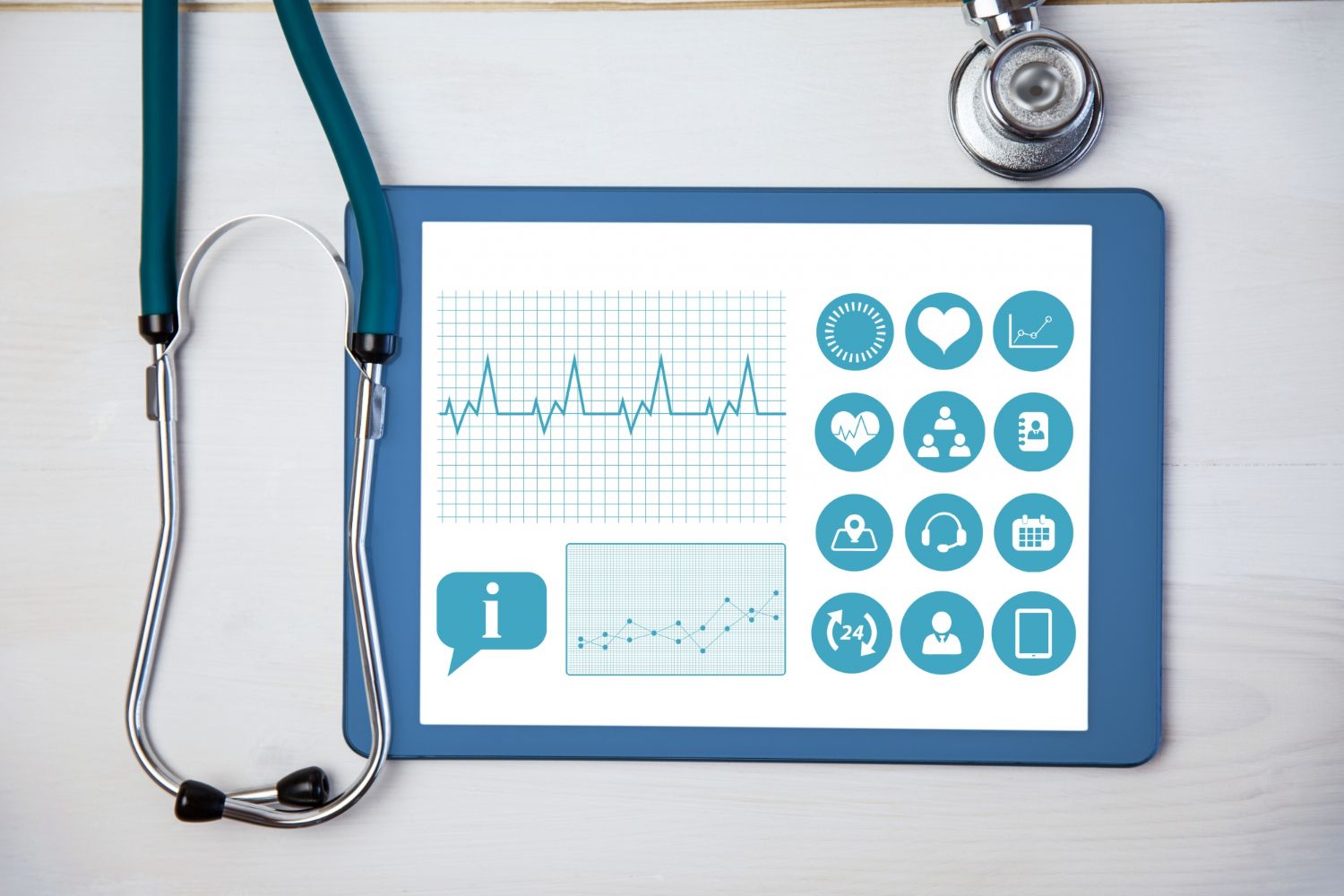
The Role of Data Science in Healthcare Analytics
Data science plays a pivotal role in healthcare analytics by leveraging advanced analytical techniques, machine learning algorithms, and artificial intelligence (AI) to extract valuable insights from healthcare data. The key components of data science in healthcare analytics include:
1. Data Collection and Integration: Data science involves collecting and integrating diverse sources of healthcare data, including structured and unstructured data from electronic health records, medical devices, wearables, and other sources. Data scientists employ data integration techniques to standardize data formats, resolve inconsistencies, and create unified datasets for analysis.
2. Data Preprocessing and Cleaning: Data preprocessing is an essential step in data science that involves cleaning, transforming, and preparing raw data for analysis. Data scientists use techniques such as data cleaning, missing value imputation, outlier detection, and feature engineering to ensure data quality and reliability before analysis.
3. Exploratory Data Analysis (EDA): Exploratory data analysis is a fundamental aspect of data science that involves visualizing and summarizing data to gain insights into patterns, trends, and relationships. Data scientists use statistical techniques, data visualization tools, and exploratory data analysis to identify patterns and anomalies in healthcare data and guide subsequent analysis.
4. Predictive Modeling and Machine Learning: Predictive modeling and machine learning are key techniques in data science that involve building mathematical models to predict outcomes or make decisions based on historical data. In healthcare analytics, data scientists develop predictive models to forecast disease risk, predict patient outcomes, identify high-risk patients, and optimize treatment strategies.
5. Natural Language Processing (NLP): Natural language processing is a branch of data science that focuses on understanding and analyzing human language. In healthcare analytics, NLP techniques are used to extract valuable information from unstructured clinical notes, medical literature, and patient records, enabling automated documentation, clinical decision support, and sentiment analysis.
6. Deep Learning and Image Analysis: Deep learning is a subset of machine learning that employs neural networks with multiple layers to extract features and patterns from complex data. In healthcare analytics, deep learning techniques are used for image analysis tasks such as medical image classification, segmentation, and object detection, enabling automated diagnosis and medical imaging interpretation.
7. Prescriptive Analytics: Prescriptive analytics is an advanced analytics technique that goes beyond descriptive and predictive analytics to recommend actions or interventions based on analysis results. In healthcare analytics, prescriptive analytics helps healthcare providers make informed decisions about treatment options, resource allocation, and care management strategies to improve patient outcomes and optimize healthcare delivery.
Applications of Data Science in Healthcare Analytics
Data science has a wide range of applications in healthcare analytics, spanning clinical, operational, and administrative domains. Some key applications include:
- Clinical Decision Support: Data science enables the development of clinical decision support systems that provide healthcare providers with real-time insights and recommendations at the point of care. These systems leverage patient data, clinical guidelines, and evidence-based research to assist clinicians in making informed decisions about diagnosis, treatment, and care management.
- Predictive Risk Modeling: Data science enables the development of predictive risk models that identify individuals at risk of developing specific diseases or adverse health outcomes. These models leverage patient data, demographic information, medical history, and clinical indicators to stratify patients based on their risk profiles and prioritize interventions for high-risk individuals.
- Disease Surveillance and Outbreak Detection: Data science plays a crucial role in disease surveillance and outbreak detection by analyzing epidemiological data, syndromic surveillance data, and social media data to detect early warning signs of infectious disease outbreaks and public health emergencies. These analyses help public health authorities monitor disease trends, track the spread of infectious diseases, and implement timely interventions to mitigate the impact of outbreaks.
- Healthcare Operations Optimization: Data science enables healthcare organizations to optimize their operations and resource allocation by analyzing data on patient flow, bed occupancy, staffing levels, and supply chain management. These analyses help healthcare providers improve efficiency, reduce wait times, and enhance patient satisfaction by optimizing the allocation of resources and improving workflow processes.
- Personalized Medicine and Precision Health: Data science enables personalized medicine and precision health initiatives by analyzing genomic data, clinical biomarkers, and patient characteristics to tailor treatment plans and interventions to individual patients. These analyses help healthcare providers deliver more effective and targeted therapies, reduce adverse drug reactions, and improve patient outcomes by optimizing treatment selection and dosing.
Benefits of Data Science in Healthcare Analytics
The integration of data science into healthcare analytics offers numerous benefits for healthcare providers, patients, and stakeholders, including:
- Improved Clinical Outcomes: Data science enables healthcare providers to leverage data-driven insights to improve clinical decision-making, optimize treatment strategies, and enhance patient outcomes. By identifying high-risk patients, predicting disease progression, and recommending personalized interventions, data science empowers healthcare providers to deliver more effective and timely care.
- Enhanced Patient Care: Data science facilitates the delivery of personalized and patient-centered care by tailoring treatment plans, interventions, and care pathways to individual patient needs and preferences. By analyzing patient data, monitoring health trends, and predicting future health risks, data science helps healthcare providers proactively manage chronic conditions, prevent complications, and improve overall patient well-being.
- Operational Efficiency: Data science drives operational efficiency and cost savings by optimizing resource allocation, streamlining workflow processes, and reducing unnecessary interventions and hospital readmissions. By analyzing healthcare data, identifying bottlenecks, and optimizing scheduling and staffing levels, data science helps healthcare organizations improve efficiency, reduce waste, and enhance productivity.
- Enhanced Population Health Management: Data science enables population health management initiatives by analyzing population-level data, identifying at-risk populations, and implementing targeted interventions to improve health outcomes and reduce disparities. By stratifying populations based on risk profiles, predicting disease prevalence, and identifying social determinants of health, data science supports population health initiatives aimed at improving the health and well-being of entire communities.
- Data-Driven Decision-Making: Data science empowers healthcare providers, policymakers, and administrators to make data-driven decisions based on evidence, insights, and predictive analytics. By providing actionable insights, visualizations, and dashboards, data science helps stakeholders understand healthcare trends, identify areas for improvement, and implement effective interventions to drive positive change and innovation in healthcare delivery and management.
Challenges and Considerations
While data science holds tremendous promise for transforming healthcare analytics, it also presents several challenges and considerations that must be addressed:
- Data Quality and Integration: Ensuring the quality, accuracy, and reliability of healthcare data is essential for generating meaningful insights and making informed decisions. Data scientists must address challenges related to data silos, interoperability, and data governance to integrate disparate datasets and ensure data consistency and completeness for analysis.
- Privacy and Security: Protecting patient privacy and maintaining data security are paramount in healthcare analytics to comply with regulatory requirements such as HIPAA and GDPR. Data scientists must implement robust security measures, encryption techniques, and access controls to safeguard sensitive healthcare data from unauthorized access, breaches, and cyberattacks. Additionally, they must adhere to ethical guidelines and privacy regulations when handling patient information to protect patient confidentiality and trust.
- Interpretability and Transparency: Ensuring the interpretability and transparency of data science models and algorithms is critical for gaining stakeholder trust and understanding the rationale behind model predictions and recommendations. Data scientists must employ interpretable machine learning techniques, explainable AI models, and transparent decision-making processes to enhance model explainability and accountability.
- Bias and Fairness: Addressing bias and fairness in healthcare analytics is essential to prevent algorithmic discrimination and ensure equitable access to healthcare services and resources. Data scientists must identify and mitigate bias in datasets, algorithms, and decision-making processes to avoid perpetuating existing disparities and biases in healthcare delivery and outcomes.
- Regulatory Compliance: Navigating regulatory compliance requirements, including HIPAA, GDPR, and FDA regulations, is a significant challenge in healthcare analytics. Data scientists must stay informed about changes in regulations, guidelines, and enforcement actions to ensure compliance with data privacy, security, and ethical standards while conducting research and analysis.
- Data Governance and Ethics: Establishing robust data governance frameworks and ethical guidelines is essential for responsible data science practices in healthcare analytics. Data scientists must adhere to principles of data stewardship, transparency, and accountability and uphold ethical standards when collecting, analyzing, and sharing healthcare data to protect patient rights and privacy.
- Resource Constraints: Overcoming resource constraints, including limited access to data, expertise, and computational resources, poses challenges for healthcare organizations implementing data science initiatives. Data scientists must collaborate with interdisciplinary teams, leverage open-source tools and platforms, and invest in training and capacity building to overcome resource limitations and maximize the impact of data science in healthcare analytics.
Future Directions and Opportunities
Despite the challenges, data science holds immense potential for driving innovation and improving healthcare outcomes in the future. Some key future directions and opportunities for data science in healthcare analytics include:
- Advanced Analytics and Predictive Modeling: Advancing predictive modeling techniques and machine learning algorithms to predict disease trajectories, treatment responses, and healthcare utilization patterns with higher accuracy and precision.
- Real-Time Analytics and Decision Support: Developing real-time analytics platforms and clinical decision support systems that provide actionable insights and recommendations to healthcare providers at the point of care to improve clinical outcomes and patient safety.
- Precision Medicine and Personalized Healthcare: Expanding precision medicine initiatives and personalized healthcare approaches that leverage genomic data, biomarkers, and patient characteristics to tailor treatment plans and interventions to individual patients' unique needs and preferences.
- Population Health Management and Public Health: Enhancing population health management strategies and public health interventions through data-driven approaches that address social determinants of health, health disparities, and community-level factors impacting health outcomes.
- Healthcare AI and Automation: Harnessing the power of artificial intelligence and automation technologies to automate routine tasks, streamline healthcare workflows, and augment clinical decision-making processes to improve efficiency and reduce healthcare costs.
- Interoperability and Data Sharing: Promoting interoperability and data sharing initiatives that enable seamless exchange of healthcare data across systems, organizations, and geographic boundaries to support collaborative research, quality improvement initiatives, and public health surveillance efforts.
Conclusion
In conclusion, data science plays a pivotal role in healthcare analytics by leveraging advanced analytical techniques, machine learning algorithms, and artificial intelligence to extract valuable insights from healthcare data. By harnessing the power of data science, healthcare organizations can enhance decision-making, improve patient care, and optimize operational efficiency to drive positive outcomes and innovation in healthcare delivery and management. However, addressing challenges related to data quality, privacy, bias, and regulatory compliance is essential to realizing the full potential of data science in healthcare analytics. With ongoing advancements in technology and collaboration across interdisciplinary teams, the future of data science in healthcare holds tremendous promise for transforming the way we deliver and experience healthcare services.